An open source library for building end-to-end dialog systems and training chatbots.
Project description
[](https://github.com/deepmipt/DeepPavlov/blob/master/LICENSE)

**We are in a really early Alpha release. You should be ready for hard adventures.
If you have updated to version 0.0.2 or greater - please re-download all pre-trained models**
DeepPavlov is an open-source conversational AI library built on [TensorFlow](https://www.tensorflow.org/) and [Keras](https://keras.io/). It is designed for
* development of production ready chat-bots and complex conversational systems,
* NLP and dialog systems research.
Our goal is to enable AI-application developers and researchers with:
* set of pre-trained NLP models, pre-defined dialog system components (ML/DL/Rule-based) and pipeline templates;
* a framework for implementing and testing their own dialog models;
* tools for application integration with adjacent infrastructure (messengers, helpdesk software etc.);
* benchmarking environment for conversational models and uniform access to relevant datasets.
# Demo
Demo of selected features is available at [demo.ipavlov.ai](https://demo.ipavlov.ai/)
# Conceptual overview
<!-- ### Principles
The library is designed according to the following principles:
* hybrid ML/DL/Rule-based architecture as a current approach
* support of modular dialog system design
* end-to-end deep learning architecture as a long-term goal
* component-based software engineering, maximization of reusability
* multiple alternative solutions for the same NLP task to enable flexible data-driven configuration
* easy extension and benchmarking -->
<!-- ### Target Architecture
Target architecture of our library: -->
<p align="left">
<img src="dp_agnt_diag.png"/>
</p>
## Key Concepts
* `Agent` is a conversational agent communicating with users in natural language (text).
* `Skill` fulfills user’s goal in some domain. Typically, this is accomplished by presenting information or completing transaction (e.g. answer question by FAQ, booking tickets etc.). However, for some tasks a success of interaction is defined as continuous engagement (e.g. chit-chat).
* `Model` is a reusable functional component of `Skill`.
* `Rule-based Models` cannot be trained.
* `Machine Learning Models` can be trained only stand alone.
* `Deep Learning Models` can be trained independently and in an end-to-end mode being joined in a chain.
* `Skill Manager` performs selection of the `Skill` to generate response.
* ` Chainer` builds an agent/component pipeline from heterogeneous components (rule-based/ml/dl). It allows to train and infer models in a pipeline as a whole.
The smallest building block of the library is `Model`. `Model` stands for any kind of function in an NLP pipeline. It can be implemented as a neural network, a non-neural ML model or a rule-based system. Besides that, `Model` can have nested structure, i.e. a `Model` can include other `Model`'(s).
`Model`s can be joined into a `Skill`. `Skill` solves a larger NLP task compared to `Model`. However, in terms of implementation `Skill`s are not different from `Model`s. The only restriction of `Skill`s is that their input and output should both be strings. Therefore, `Skill`s are usually associated with dialogue tasks.
`Agent` is supposed to be a multi-purpose dialogue system that comprises several `Skill`s and can switch between them. It can be a dialogue system that contains a goal-oriented and chatbot skills and chooses which one to use for generating the answer depending on user input.
DeepPavlov is built on top of machine learning frameworks [TensorFlow](https://www.tensorflow.org/) and [Keras](https://keras.io/). Other external libraries can be used to build basic components.
---
# Installation
0. Currently we support only `Linux` platform and `Python 3.6` (**`Python 3.5` is not supported!**)
1. Create a virtual environment with `Python 3.6`
```
virtualenv env
```
2. Activate the environment.
```
source ./env/bin/activate
```
3. Clone the repo and `cd` to project root
```
git clone https://github.com/deepmipt/DeepPavlov.git
cd DeepPavlov
```
4. Install the requirements:
```
python setup.py develop
```
5. Install `spacy` dependencies:
```
python -m spacy download en
```
# Quick start
To use our pre-trained models, you should first download them:
```
python -m deeppavlov.deep download <path_to_config>
```
or you can use additional key `-d` to automatically download all required models and data with any command like `interact`, `riseapi`, etc.
Then you can interact with the models or train them with the following command:
```
python -m deeppavlov.deep <mode> <path_to_config> [-d]
```
* `<mode>` can be `train`, `predict`, `interact`, `interactbot` or `riseapi`
* `<path_to_config>` should be a path to an NLP pipeline json config (e.g. `deeppavlov/configs/ner/slotfill_dstc2.json`)
or a name without the `.json` extension of one of the config files [provided](deeppavlov/configs) in this repository (e.g. `slotfill_dstc2`)
For the `interactbot` mode you should specify Telegram bot token in `-t` parameter or in `TELEGRAM_TOKEN` environment variable. Also if you want to get custom `/start` and `/help` Telegram messages for the running model you should:
* Add section to `utils/telegram_utils/model_info.json` with your custom Telegram messages
* In model config file specify `metadata.labels.telegram_utils` parameter with name which refers to the added section of `utils/telegram_utils/model_info.json`
For `riseapi` mode you should specify api settings (host, port, etc.) in [*utils/server_utils/server_config.json*](utils/server_utils/server_config.json) configuration file. If provided, values from *model_defaults* section override values for the same parameters from *common_defaults* section. Model names in *model_defaults* section should be similar to the class names of the models main component.
For `predict` you can specify path to input file with `-f` or `--input-file` parameter, otherwise, data will be taken
from stdin.
Every line of input text will be used as a pipeline input parameter, so one example will consist of as many lines,
as many input parameters your pipeline expects.
You can also specify batch size with `-b` or `--batch-size` parameter.
Available model configs are:
- ```deeppavlov/configs/go_bot/*.json```
- ```deeppavlov/configs/seq2seq_go_bot/*.json```
- ```deeppavlov/configs/odqa/*.json```
- ```deeppavlov/configs/squad/*.json```
- ```deeppavlov/configs/intents/*.json```
- ```deeppavlov/configs/ner/*.json```
- ```deeppavlov/configs/ranking/*.json```
- ```deeppavlov/configs/spelling_correction/*.json```
# Features
| Component | Description |
| --------- | ----------- |
| [NER component](deeppavlov/models/ner/README.md) | Based on neural Named Entity Recognition network. The NER component reproduces architecture from the paper [Application of a Hybrid Bi-LSTM-CRF model to the task of Russian Named Entity Recognition](https://arxiv.org/pdf/1709.09686.pdf) which is inspired by Bi-LSTM+CRF architecture from https://arxiv.org/pdf/1603.01360.pdf. |
| [Slot filling components](deeppavlov/models/slotfill/README.md) | Based on fuzzy Levenshtein search to extract normalized slot values from text. The components either rely on NER results or perform needle in haystack search.|
| [Intent classification component](deeppavlov/models/classifiers/intents/README.md) | Based on shallow-and-wide Convolutional Neural Network architecture from [Kim Y. Convolutional neural networks for sentence classification – 2014](https://arxiv.org/pdf/1408.5882). The model allows multilabel classification of sentences. |
| [Automatic spelling correction component](deeppavlov/models/spelling_correction/README.md) | Pipelines that use candidates search in a static dictionary and an ARPA language model to correct spelling errors. |
| [Ranking component](deeppavlov/models/ranking/README.md) | Based on [LSTM-based deep learning models for non-factoid answer selection](https://arxiv.org/abs/1511.04108). The model performs ranking of responses or contexts from some database by their relevance for the given context. |
| [Question Answering component](deeppavlov/models/squad/README.md) | Based on [R-NET: Machine Reading Comprehension with Self-matching Networks](https://www.microsoft.com/en-us/research/publication/mrc/). The model solves the task of looking for an answer on a question in a given context ([SQuAD](https://rajpurkar.github.io/SQuAD-explorer/) task format). |
| [Morphological tagging component](deeppavlov/models/morpho_tagger/README.md) | Based on character-based approach to morphological tagging [Heigold et al., 2017. An extensive empirical evaluation of character-based morphological tagging for 14 languages](http://www.aclweb.org/anthology/E17-1048). A state-of-the-art model for Russian and several other languages. Model assigns morphological tags in UD format to sequences of words.|
| **Skills** | |
| [Goal-oriented bot](deeppavlov/skills/go_bot/README.md) | Based on Hybrid Code Networks (HCNs) architecture from [Jason D. Williams, Kavosh Asadi, Geoffrey Zweig, Hybrid Code Networks: practical and efficient end-to-end dialog control with supervised and reinforcement learning – 2017](https://arxiv.org/abs/1702.03274). It allows to predict responses in goal-oriented dialog. The model is customizable: embeddings, slot filler and intent classifier can switched on and off on demand. |
| [Seq2seq goal-oriented bot](deeppavlov/skills/seq2seq_go_bot/README.md) | Dialogue agent predicts responses in a goal-oriented dialog and is able to handle multiple domains (pretrained bot allows calendar scheduling, weather information retrieval, and point-of-interest navigation). The model is end-to-end differentiable and does not need to explicitly model dialogue state or belief trackers. |
|[ODQA](deeppavlov/skills/odqa/README.md) | An open domain question answering skill. The skill accepts free-form questions about the world and outputs an answer based on its Wikipedia knowledge.|
| **Embeddings** | |
| [Pre-trained embeddings for the Russian language](pretrained-vectors.md) | Word vectors for the Russian language trained on joint [Russian Wikipedia](https://ru.wikipedia.org/wiki/%D0%97%D0%B0%D0%B3%D0%BB%D0%B0%D0%B2%D0%BD%D0%B0%D1%8F_%D1%81%D1%82%D1%80%D0%B0%D0%BD%D0%B8%D1%86%D0%B0) and [Lenta.ru](https://lenta.ru/) corpora. |
# Basic examples
View video demo of deployment of a goal-oriented bot and a slot-filling model with Telegram UI
[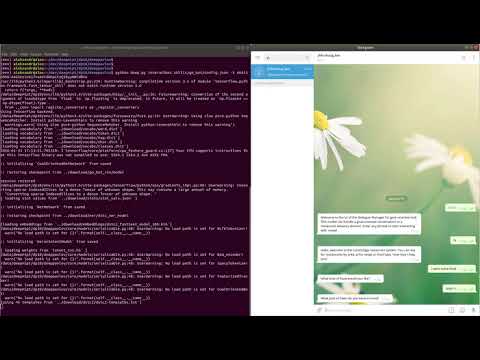](https://youtu.be/yzoiCa_sMuY)
* Run goal-oriented bot with Telegram interface:
```
python -m deeppavlov.deep interactbot deeppavlov/configs/go_bot/gobot_dstc2.json -d -t <TELEGRAM_TOKEN>
```
* Run goal-oriented bot with console interface:
```
python -m deeppavlov.deep interact deeppavlov/configs/go_bot/gobot_dstc2.json -d
```
* Run goal-oriented bot with REST API:
```
python -m deeppavlov.deep riseapi deeppavlov/configs/go_bot/gobot_dstc2.json -d
```
* Run slot-filling model with Telegram interface:
```
python -m deeppavlov.deep interactbot deeppavlov/configs/ner/slotfill_dstc2.json -d -t <TELEGRAM_TOKEN>
```
* Run slot-filling model with console interface:
```
python -m deeppavlov.deep interact deeppavlov/configs/ner/slotfill_dstc2.json -d
```
* Run slot-filling model with REST API:
```
python -m deeppavlov.deep riseapi deeppavlov/configs/ner/slotfill_dstc2.json -d
```
* Predict intents on every line in a file:
```
python -m deeppavlov.deep predict deeppavlov/configs/intents/intents_snips.json -d --batch-size 15 < /data/in.txt > /data/out.txt
```
---
# Technical overview
## Project modules
<table>
<tr>
<td><b> deeppavlov.core.commands </b></td>
<td> basic training and inference functions </td>
</tr>
<tr>
<td><b> deeppavlov.core.common </b></td>
<td> registration and classes initialization functionality, class method decorators </td>
</tr>
<tr>
<td><b> deeppavlov.core.data </b></td>
<td> basic <b><i>DatasetIterator</i></b>, <b><i>DatasetReader</i></b> and <b><i>Vocab</i></b> classes </td>
</tr>
<tr>
<td><b> deeppavlov.core.layers </b></td>
<td> collection of commonly used <b><i>Layers</i></b> for TF models </td>
</tr>
<tr>
<td><b> deeppavlov.core.models </b></td>
<td> abstract model classes and interfaces </td>
</tr>
<tr>
<td><b> deeppavlov.dataset_readers </b></td>
<td> concrete <b><i>DatasetReader</i></b> classes </td>
</tr>
<tr>
<td><b> deeppavlov.dataset_iterators </b></td>
<td> concrete <b><i>DatasetIterators</i></b> classes </td>
</tr>
<tr>
<td><b> deeppavlov.metrics </b></td>
<td> different <b><i>Metric</i></b> functions </td>
</tr>
<tr>
<td><b> deeppavlov.models </b></td>
<td> concrete <b><i>Model</i></b> classes </td>
</tr>
<tr>
<td><b> deeppavlov.skills </b></td>
<td> <b><i>Skill</i></b> classes. Skills are dialog models.</td>
</tr>
<tr>
<td><b> deeppavlov.vocabs </b></td>
<td> concrete <b><i>Vocab</i></b> classes </td>
</tr>
</table>
## Config
An NLP pipeline config is a JSON file that contains one required element `chainer`:
```
{
"chainer": {
"in": ["x"],
"in_y": ["y"],
"pipe": [
...
],
"out": ["y_predicted"]
}
}
```
Chainer is a core concept of DeepPavlov library: chainer builds a pipeline from heterogeneous components
(rule-based/ml/dl) and allows to train or infer from pipeline as a whole. Each component in the pipeline specifies
its inputs and outputs as arrays of names, for example: `"in": ["tokens", "features"]` and `"out": ["token_embeddings", "features_embeddings"]` and you can chain outputs of one components with inputs of other components:
```json
{
"class": "deeppavlov.models.preproccessors.str_lower:StrLower",
"in": ["x"],
"out": ["x_lower"]
},
{
"name": "nltk_tokenizer",
"in": ["x_lower"],
"out": ["x_tokens"]
},
```
Each [Component](deeppavlov/core/models/component.py) in the pipeline must implement method `__call__` and has `name` parameter, which is its registered codename,
or `class` parameter in the form of `module_name:ClassName`.
It can also have any other parameters which repeat its `__init__()` method arguments.
Default values of `__init__()` arguments will be overridden with the config values during the initialization of a class instance.
You can reuse components in the pipeline to process different parts of data with the help of `id` and `ref` parameters:
```json
{
"name": "nltk_tokenizer",
"id": "tokenizer",
"in": ["x_lower"],
"out": ["x_tokens"]
},
{
"ref": "tokenizer",
"in": ["y"],
"out": ["y_tokens"]
},
```
## Training
There are two abstract classes for trainable components: **Estimator** and **NNModel**.
[**Estimators**](deeppavlov/core/models/estimator.py) are fit once on any data with no batching or early stopping,
so it can be safely done at the time of pipeline initialization. `fit` method has to be implemented for each Estimator. An example of Estimator is [Vocab](deeppavlov/core/data/vocab.py).
[**NNModel**](deeppavlov/core/models/nn_model.py) requires more complex training. It can only be trained in a supervised mode (as opposed to **Estimator** which can be trained in both supervised and unsupervised settings). This process takes multiple epochs with periodic validation and logging.
`train_on_batch` method has to be implemented for each NNModel.
Training is triggered by `deeppavlov.core.commands.train.train_model_from_config()` function.
## Train config
Estimators that are trained should also have `fit_on` parameter which contains a list of input parameter names.
An NNModel should have the `in_y` parameter which contains a list of ground truth answer names. For example:
```json
[
{
"id": "classes_vocab",
"name": "default_vocab",
"fit_on": ["y"],
"level": "token",
"save_path": "vocabs/classes.dict",
"load_path": "vocabs/classes.dict"
},
{
"in": ["x"],
"in_y": ["y"],
"out": ["y_predicted"],
"name": "intent_model",
"save_path": "intents/intent_cnn",
"load_path": "intents/intent_cnn",
"classes_vocab": {
"ref": "classes_vocab"
}
}
]
```
The config for training the pipeline should have three additional elements: `dataset_reader`, `dataset_iterator` and `train`:
```
{
"dataset_reader": {
"name": ...,
...
}
"dataset_iterator": {
"name": ...,
...
},
"chainer": {
...
}
"train": {
...
}
}
```
Simplified version of trainig pipeline contains two elemens: `dataset` and `train`. The `dataset` element currently
can be used for train from classification data in `csv` and `json` formats. You can find complete examples of how to use simplified training pipeline in [intents_sample_csv.json](deeppavlov/configs/intents/intents_sample_csv.json) and [intents_sample_json.json](deeppavlov/configs/intents/intents_sample_json.json) config files.
## Train Parameters
* `epochs` — maximum number of epochs to train NNModel, defaults to `-1` (infinite)
* `batch_size`,
* `metrics` — list of names of [registered metrics](deeppavlov/metrics) to evaluate the model. The first metric in the list
is used for early stopping
* `metric_optimization` — `maximize` or `minimize` a metric, defaults to `maximize`
* `validation_patience` — how many times in a row the validation metric has to not improve for early stopping, defaults to `5`
* `val_every_n_epochs` — how often to validate the pipe, defaults to `-1` (never)
* `log_every_n_batches`, `log_every_n_epochs` — how often to calculate metrics for train data, defaults to `-1` (never)
* `validate_best`, `test_best` flags to infer the best saved model on valid and test data, defaults to `true`
## DatasetReader
`DatasetReader` class reads data and returns it in a specified format.
A concrete `DatasetReader` class should be inherited from the base
`deeppavlov.data.dataset_reader.DatasetReader` class and registered with a codename:
```python
from deeppavlov.core.common.registry import register
from deeppavlov.core.data.dataset_reader import DatasetReader
@register('dstc2_datasetreader')
class DSTC2DatasetReader(DatasetReader):
```
## DatasetIterator
`DatasetIterator` forms the sets of data ('train', 'valid', 'test') needed for training/inference and divides it into batches.
A concrete `DatasetIterator` class should be registered and can be inherited from
`deeppavlov.data.dataset_iterator.BasicDatasetIterator` class. `deeppavlov.data.dataset_iterator.BasicDatasetIterator`
is not an abstract class and can be used as a `DatasetIterator` as well.
## Inference
All components inherited from `deeppavlov.core.models.component.Component` abstract class can be used for inference. The `__call__()` method should return standard output of a component. For example, a *tokenizer* should return
*tokens*, a *NER recognizer* should return *recognized entities*, a *bot* should return an *utterance*.
A particular format of returned data should be defined in `__call__()`.
Inference is triggered by `deeppavlov.core.commands.infer.interact_model()` function. There is no need in a separate JSON for inference.
## Rest API
Each library component or skill can be easily made available for inference as a REST web service. The general method is:
`python -m deeppavlov.deep riseapi <config_path> [-d]`
(optional `-d` key is for dependencies download before service start)
Web service properties (host, port, model endpoint, GET request arguments) are provided in `utils/server_utils/server_config.json`.
Properties from `common_defaults` section are used by default unless they are overridden by component-specific properties, provided in `model_defaults` section of the `server_config.json`.
Component-specific properties are bound to the component by `server_utils` label in `metadata/labels` section of the component config. Value of `server_utils` label from component config should match with properties key from `model_defaults` section of `server_config.json`.
For example, `metadata/labels/server_utils` tag from `go_bot/gobot_dstc2.json` references to the *GoalOrientedBot* section of `server_config.json`. Therefore, `model_endpoint` parameter in `common_defaults` will be will be overridden with the same parameter from `model_defaults/GoalOrientedBot`.
Model argument names are provided as list in `model_args_names` parameter, where arguments order corresponds to component API.
When inferencing model via REST api, JSON payload keys should match component arguments names from `model_args_names`.
Default argument name for one argument components is *"context"*.
Here are POST requests examples for some of the library components:
| Component | POST request JSON payload example |
| --------- | -------------------- |
| **One argument components** |
| NER component | {"context":"Elon Musk launched his cherry Tesla roadster to the Mars orbit"} |
| Intent classification component | {"context":"I would like to go to a restaurant with Asian cuisine this evening"} |
| Automatic spelling correction component | {"context":"errror"} |
| Ranking component | {"context":"What is the average cost of life insurance services?"} |
| (Seq2seq) Goal-oriented bot | {"context":"Hello, can you help me to find and book a restaurant this evening?"} |
| **Two arguments components** |
| Question Answering component | {"context":"After 1765, growing philosophical and political differences strained the relationship between Great Britain and its colonies.", "question":"What strained the relationship between Great Britain and its colonies?"} |
Flasgger UI for API testing is provided on `<host>:<port>/apidocs` when running a component in `riseapi` mode.
# License
DeepPavlov is Apache 2.0 - licensed.
# Support and collaboration
If you have any questions, bug reports or feature requests, please feel free to post on our [Github Issues](https://github.com/deepmipt/DeepPavlov/issues) page. Please tag your issue with `bug`, `feature request`, or `question`. Also we’ll be glad to see your pull requests to add new datasets, models, embeddings, etc.
# The Team
DeepPavlov is built and maintained by [Neural Networks and Deep Learning Lab](https://mipt.ru/english/research/labs/neural-networks-and-deep-learning-lab) at [MIPT](https://mipt.ru/english/) within [iPavlov](http://ipavlov.ai/) project (part of [National Technology Initiative](https://asi.ru/eng/nti/)) and in partnership with [Sberbank](http://www.sberbank.com/).
<p align="center">
<img src="https://ipavlov.ai/img/ipavlov_footer.png" width="50%" height="50%"/>
</p>

**We are in a really early Alpha release. You should be ready for hard adventures.
If you have updated to version 0.0.2 or greater - please re-download all pre-trained models**
DeepPavlov is an open-source conversational AI library built on [TensorFlow](https://www.tensorflow.org/) and [Keras](https://keras.io/). It is designed for
* development of production ready chat-bots and complex conversational systems,
* NLP and dialog systems research.
Our goal is to enable AI-application developers and researchers with:
* set of pre-trained NLP models, pre-defined dialog system components (ML/DL/Rule-based) and pipeline templates;
* a framework for implementing and testing their own dialog models;
* tools for application integration with adjacent infrastructure (messengers, helpdesk software etc.);
* benchmarking environment for conversational models and uniform access to relevant datasets.
# Demo
Demo of selected features is available at [demo.ipavlov.ai](https://demo.ipavlov.ai/)
# Conceptual overview
<!-- ### Principles
The library is designed according to the following principles:
* hybrid ML/DL/Rule-based architecture as a current approach
* support of modular dialog system design
* end-to-end deep learning architecture as a long-term goal
* component-based software engineering, maximization of reusability
* multiple alternative solutions for the same NLP task to enable flexible data-driven configuration
* easy extension and benchmarking -->
<!-- ### Target Architecture
Target architecture of our library: -->
<p align="left">
<img src="dp_agnt_diag.png"/>
</p>
## Key Concepts
* `Agent` is a conversational agent communicating with users in natural language (text).
* `Skill` fulfills user’s goal in some domain. Typically, this is accomplished by presenting information or completing transaction (e.g. answer question by FAQ, booking tickets etc.). However, for some tasks a success of interaction is defined as continuous engagement (e.g. chit-chat).
* `Model` is a reusable functional component of `Skill`.
* `Rule-based Models` cannot be trained.
* `Machine Learning Models` can be trained only stand alone.
* `Deep Learning Models` can be trained independently and in an end-to-end mode being joined in a chain.
* `Skill Manager` performs selection of the `Skill` to generate response.
* ` Chainer` builds an agent/component pipeline from heterogeneous components (rule-based/ml/dl). It allows to train and infer models in a pipeline as a whole.
The smallest building block of the library is `Model`. `Model` stands for any kind of function in an NLP pipeline. It can be implemented as a neural network, a non-neural ML model or a rule-based system. Besides that, `Model` can have nested structure, i.e. a `Model` can include other `Model`'(s).
`Model`s can be joined into a `Skill`. `Skill` solves a larger NLP task compared to `Model`. However, in terms of implementation `Skill`s are not different from `Model`s. The only restriction of `Skill`s is that their input and output should both be strings. Therefore, `Skill`s are usually associated with dialogue tasks.
`Agent` is supposed to be a multi-purpose dialogue system that comprises several `Skill`s and can switch between them. It can be a dialogue system that contains a goal-oriented and chatbot skills and chooses which one to use for generating the answer depending on user input.
DeepPavlov is built on top of machine learning frameworks [TensorFlow](https://www.tensorflow.org/) and [Keras](https://keras.io/). Other external libraries can be used to build basic components.
---
# Installation
0. Currently we support only `Linux` platform and `Python 3.6` (**`Python 3.5` is not supported!**)
1. Create a virtual environment with `Python 3.6`
```
virtualenv env
```
2. Activate the environment.
```
source ./env/bin/activate
```
3. Clone the repo and `cd` to project root
```
git clone https://github.com/deepmipt/DeepPavlov.git
cd DeepPavlov
```
4. Install the requirements:
```
python setup.py develop
```
5. Install `spacy` dependencies:
```
python -m spacy download en
```
# Quick start
To use our pre-trained models, you should first download them:
```
python -m deeppavlov.deep download <path_to_config>
```
or you can use additional key `-d` to automatically download all required models and data with any command like `interact`, `riseapi`, etc.
Then you can interact with the models or train them with the following command:
```
python -m deeppavlov.deep <mode> <path_to_config> [-d]
```
* `<mode>` can be `train`, `predict`, `interact`, `interactbot` or `riseapi`
* `<path_to_config>` should be a path to an NLP pipeline json config (e.g. `deeppavlov/configs/ner/slotfill_dstc2.json`)
or a name without the `.json` extension of one of the config files [provided](deeppavlov/configs) in this repository (e.g. `slotfill_dstc2`)
For the `interactbot` mode you should specify Telegram bot token in `-t` parameter or in `TELEGRAM_TOKEN` environment variable. Also if you want to get custom `/start` and `/help` Telegram messages for the running model you should:
* Add section to `utils/telegram_utils/model_info.json` with your custom Telegram messages
* In model config file specify `metadata.labels.telegram_utils` parameter with name which refers to the added section of `utils/telegram_utils/model_info.json`
For `riseapi` mode you should specify api settings (host, port, etc.) in [*utils/server_utils/server_config.json*](utils/server_utils/server_config.json) configuration file. If provided, values from *model_defaults* section override values for the same parameters from *common_defaults* section. Model names in *model_defaults* section should be similar to the class names of the models main component.
For `predict` you can specify path to input file with `-f` or `--input-file` parameter, otherwise, data will be taken
from stdin.
Every line of input text will be used as a pipeline input parameter, so one example will consist of as many lines,
as many input parameters your pipeline expects.
You can also specify batch size with `-b` or `--batch-size` parameter.
Available model configs are:
- ```deeppavlov/configs/go_bot/*.json```
- ```deeppavlov/configs/seq2seq_go_bot/*.json```
- ```deeppavlov/configs/odqa/*.json```
- ```deeppavlov/configs/squad/*.json```
- ```deeppavlov/configs/intents/*.json```
- ```deeppavlov/configs/ner/*.json```
- ```deeppavlov/configs/ranking/*.json```
- ```deeppavlov/configs/spelling_correction/*.json```
# Features
| Component | Description |
| --------- | ----------- |
| [NER component](deeppavlov/models/ner/README.md) | Based on neural Named Entity Recognition network. The NER component reproduces architecture from the paper [Application of a Hybrid Bi-LSTM-CRF model to the task of Russian Named Entity Recognition](https://arxiv.org/pdf/1709.09686.pdf) which is inspired by Bi-LSTM+CRF architecture from https://arxiv.org/pdf/1603.01360.pdf. |
| [Slot filling components](deeppavlov/models/slotfill/README.md) | Based on fuzzy Levenshtein search to extract normalized slot values from text. The components either rely on NER results or perform needle in haystack search.|
| [Intent classification component](deeppavlov/models/classifiers/intents/README.md) | Based on shallow-and-wide Convolutional Neural Network architecture from [Kim Y. Convolutional neural networks for sentence classification – 2014](https://arxiv.org/pdf/1408.5882). The model allows multilabel classification of sentences. |
| [Automatic spelling correction component](deeppavlov/models/spelling_correction/README.md) | Pipelines that use candidates search in a static dictionary and an ARPA language model to correct spelling errors. |
| [Ranking component](deeppavlov/models/ranking/README.md) | Based on [LSTM-based deep learning models for non-factoid answer selection](https://arxiv.org/abs/1511.04108). The model performs ranking of responses or contexts from some database by their relevance for the given context. |
| [Question Answering component](deeppavlov/models/squad/README.md) | Based on [R-NET: Machine Reading Comprehension with Self-matching Networks](https://www.microsoft.com/en-us/research/publication/mrc/). The model solves the task of looking for an answer on a question in a given context ([SQuAD](https://rajpurkar.github.io/SQuAD-explorer/) task format). |
| [Morphological tagging component](deeppavlov/models/morpho_tagger/README.md) | Based on character-based approach to morphological tagging [Heigold et al., 2017. An extensive empirical evaluation of character-based morphological tagging for 14 languages](http://www.aclweb.org/anthology/E17-1048). A state-of-the-art model for Russian and several other languages. Model assigns morphological tags in UD format to sequences of words.|
| **Skills** | |
| [Goal-oriented bot](deeppavlov/skills/go_bot/README.md) | Based on Hybrid Code Networks (HCNs) architecture from [Jason D. Williams, Kavosh Asadi, Geoffrey Zweig, Hybrid Code Networks: practical and efficient end-to-end dialog control with supervised and reinforcement learning – 2017](https://arxiv.org/abs/1702.03274). It allows to predict responses in goal-oriented dialog. The model is customizable: embeddings, slot filler and intent classifier can switched on and off on demand. |
| [Seq2seq goal-oriented bot](deeppavlov/skills/seq2seq_go_bot/README.md) | Dialogue agent predicts responses in a goal-oriented dialog and is able to handle multiple domains (pretrained bot allows calendar scheduling, weather information retrieval, and point-of-interest navigation). The model is end-to-end differentiable and does not need to explicitly model dialogue state or belief trackers. |
|[ODQA](deeppavlov/skills/odqa/README.md) | An open domain question answering skill. The skill accepts free-form questions about the world and outputs an answer based on its Wikipedia knowledge.|
| **Embeddings** | |
| [Pre-trained embeddings for the Russian language](pretrained-vectors.md) | Word vectors for the Russian language trained on joint [Russian Wikipedia](https://ru.wikipedia.org/wiki/%D0%97%D0%B0%D0%B3%D0%BB%D0%B0%D0%B2%D0%BD%D0%B0%D1%8F_%D1%81%D1%82%D1%80%D0%B0%D0%BD%D0%B8%D1%86%D0%B0) and [Lenta.ru](https://lenta.ru/) corpora. |
# Basic examples
View video demo of deployment of a goal-oriented bot and a slot-filling model with Telegram UI
[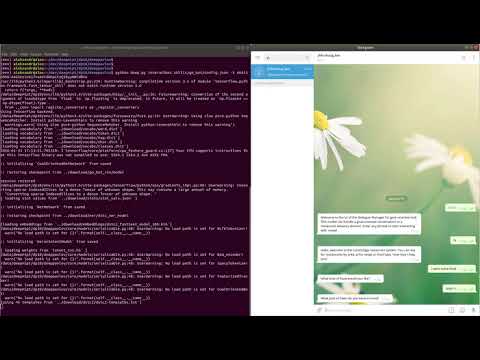](https://youtu.be/yzoiCa_sMuY)
* Run goal-oriented bot with Telegram interface:
```
python -m deeppavlov.deep interactbot deeppavlov/configs/go_bot/gobot_dstc2.json -d -t <TELEGRAM_TOKEN>
```
* Run goal-oriented bot with console interface:
```
python -m deeppavlov.deep interact deeppavlov/configs/go_bot/gobot_dstc2.json -d
```
* Run goal-oriented bot with REST API:
```
python -m deeppavlov.deep riseapi deeppavlov/configs/go_bot/gobot_dstc2.json -d
```
* Run slot-filling model with Telegram interface:
```
python -m deeppavlov.deep interactbot deeppavlov/configs/ner/slotfill_dstc2.json -d -t <TELEGRAM_TOKEN>
```
* Run slot-filling model with console interface:
```
python -m deeppavlov.deep interact deeppavlov/configs/ner/slotfill_dstc2.json -d
```
* Run slot-filling model with REST API:
```
python -m deeppavlov.deep riseapi deeppavlov/configs/ner/slotfill_dstc2.json -d
```
* Predict intents on every line in a file:
```
python -m deeppavlov.deep predict deeppavlov/configs/intents/intents_snips.json -d --batch-size 15 < /data/in.txt > /data/out.txt
```
---
# Technical overview
## Project modules
<table>
<tr>
<td><b> deeppavlov.core.commands </b></td>
<td> basic training and inference functions </td>
</tr>
<tr>
<td><b> deeppavlov.core.common </b></td>
<td> registration and classes initialization functionality, class method decorators </td>
</tr>
<tr>
<td><b> deeppavlov.core.data </b></td>
<td> basic <b><i>DatasetIterator</i></b>, <b><i>DatasetReader</i></b> and <b><i>Vocab</i></b> classes </td>
</tr>
<tr>
<td><b> deeppavlov.core.layers </b></td>
<td> collection of commonly used <b><i>Layers</i></b> for TF models </td>
</tr>
<tr>
<td><b> deeppavlov.core.models </b></td>
<td> abstract model classes and interfaces </td>
</tr>
<tr>
<td><b> deeppavlov.dataset_readers </b></td>
<td> concrete <b><i>DatasetReader</i></b> classes </td>
</tr>
<tr>
<td><b> deeppavlov.dataset_iterators </b></td>
<td> concrete <b><i>DatasetIterators</i></b> classes </td>
</tr>
<tr>
<td><b> deeppavlov.metrics </b></td>
<td> different <b><i>Metric</i></b> functions </td>
</tr>
<tr>
<td><b> deeppavlov.models </b></td>
<td> concrete <b><i>Model</i></b> classes </td>
</tr>
<tr>
<td><b> deeppavlov.skills </b></td>
<td> <b><i>Skill</i></b> classes. Skills are dialog models.</td>
</tr>
<tr>
<td><b> deeppavlov.vocabs </b></td>
<td> concrete <b><i>Vocab</i></b> classes </td>
</tr>
</table>
## Config
An NLP pipeline config is a JSON file that contains one required element `chainer`:
```
{
"chainer": {
"in": ["x"],
"in_y": ["y"],
"pipe": [
...
],
"out": ["y_predicted"]
}
}
```
Chainer is a core concept of DeepPavlov library: chainer builds a pipeline from heterogeneous components
(rule-based/ml/dl) and allows to train or infer from pipeline as a whole. Each component in the pipeline specifies
its inputs and outputs as arrays of names, for example: `"in": ["tokens", "features"]` and `"out": ["token_embeddings", "features_embeddings"]` and you can chain outputs of one components with inputs of other components:
```json
{
"class": "deeppavlov.models.preproccessors.str_lower:StrLower",
"in": ["x"],
"out": ["x_lower"]
},
{
"name": "nltk_tokenizer",
"in": ["x_lower"],
"out": ["x_tokens"]
},
```
Each [Component](deeppavlov/core/models/component.py) in the pipeline must implement method `__call__` and has `name` parameter, which is its registered codename,
or `class` parameter in the form of `module_name:ClassName`.
It can also have any other parameters which repeat its `__init__()` method arguments.
Default values of `__init__()` arguments will be overridden with the config values during the initialization of a class instance.
You can reuse components in the pipeline to process different parts of data with the help of `id` and `ref` parameters:
```json
{
"name": "nltk_tokenizer",
"id": "tokenizer",
"in": ["x_lower"],
"out": ["x_tokens"]
},
{
"ref": "tokenizer",
"in": ["y"],
"out": ["y_tokens"]
},
```
## Training
There are two abstract classes for trainable components: **Estimator** and **NNModel**.
[**Estimators**](deeppavlov/core/models/estimator.py) are fit once on any data with no batching or early stopping,
so it can be safely done at the time of pipeline initialization. `fit` method has to be implemented for each Estimator. An example of Estimator is [Vocab](deeppavlov/core/data/vocab.py).
[**NNModel**](deeppavlov/core/models/nn_model.py) requires more complex training. It can only be trained in a supervised mode (as opposed to **Estimator** which can be trained in both supervised and unsupervised settings). This process takes multiple epochs with periodic validation and logging.
`train_on_batch` method has to be implemented for each NNModel.
Training is triggered by `deeppavlov.core.commands.train.train_model_from_config()` function.
## Train config
Estimators that are trained should also have `fit_on` parameter which contains a list of input parameter names.
An NNModel should have the `in_y` parameter which contains a list of ground truth answer names. For example:
```json
[
{
"id": "classes_vocab",
"name": "default_vocab",
"fit_on": ["y"],
"level": "token",
"save_path": "vocabs/classes.dict",
"load_path": "vocabs/classes.dict"
},
{
"in": ["x"],
"in_y": ["y"],
"out": ["y_predicted"],
"name": "intent_model",
"save_path": "intents/intent_cnn",
"load_path": "intents/intent_cnn",
"classes_vocab": {
"ref": "classes_vocab"
}
}
]
```
The config for training the pipeline should have three additional elements: `dataset_reader`, `dataset_iterator` and `train`:
```
{
"dataset_reader": {
"name": ...,
...
}
"dataset_iterator": {
"name": ...,
...
},
"chainer": {
...
}
"train": {
...
}
}
```
Simplified version of trainig pipeline contains two elemens: `dataset` and `train`. The `dataset` element currently
can be used for train from classification data in `csv` and `json` formats. You can find complete examples of how to use simplified training pipeline in [intents_sample_csv.json](deeppavlov/configs/intents/intents_sample_csv.json) and [intents_sample_json.json](deeppavlov/configs/intents/intents_sample_json.json) config files.
## Train Parameters
* `epochs` — maximum number of epochs to train NNModel, defaults to `-1` (infinite)
* `batch_size`,
* `metrics` — list of names of [registered metrics](deeppavlov/metrics) to evaluate the model. The first metric in the list
is used for early stopping
* `metric_optimization` — `maximize` or `minimize` a metric, defaults to `maximize`
* `validation_patience` — how many times in a row the validation metric has to not improve for early stopping, defaults to `5`
* `val_every_n_epochs` — how often to validate the pipe, defaults to `-1` (never)
* `log_every_n_batches`, `log_every_n_epochs` — how often to calculate metrics for train data, defaults to `-1` (never)
* `validate_best`, `test_best` flags to infer the best saved model on valid and test data, defaults to `true`
## DatasetReader
`DatasetReader` class reads data and returns it in a specified format.
A concrete `DatasetReader` class should be inherited from the base
`deeppavlov.data.dataset_reader.DatasetReader` class and registered with a codename:
```python
from deeppavlov.core.common.registry import register
from deeppavlov.core.data.dataset_reader import DatasetReader
@register('dstc2_datasetreader')
class DSTC2DatasetReader(DatasetReader):
```
## DatasetIterator
`DatasetIterator` forms the sets of data ('train', 'valid', 'test') needed for training/inference and divides it into batches.
A concrete `DatasetIterator` class should be registered and can be inherited from
`deeppavlov.data.dataset_iterator.BasicDatasetIterator` class. `deeppavlov.data.dataset_iterator.BasicDatasetIterator`
is not an abstract class and can be used as a `DatasetIterator` as well.
## Inference
All components inherited from `deeppavlov.core.models.component.Component` abstract class can be used for inference. The `__call__()` method should return standard output of a component. For example, a *tokenizer* should return
*tokens*, a *NER recognizer* should return *recognized entities*, a *bot* should return an *utterance*.
A particular format of returned data should be defined in `__call__()`.
Inference is triggered by `deeppavlov.core.commands.infer.interact_model()` function. There is no need in a separate JSON for inference.
## Rest API
Each library component or skill can be easily made available for inference as a REST web service. The general method is:
`python -m deeppavlov.deep riseapi <config_path> [-d]`
(optional `-d` key is for dependencies download before service start)
Web service properties (host, port, model endpoint, GET request arguments) are provided in `utils/server_utils/server_config.json`.
Properties from `common_defaults` section are used by default unless they are overridden by component-specific properties, provided in `model_defaults` section of the `server_config.json`.
Component-specific properties are bound to the component by `server_utils` label in `metadata/labels` section of the component config. Value of `server_utils` label from component config should match with properties key from `model_defaults` section of `server_config.json`.
For example, `metadata/labels/server_utils` tag from `go_bot/gobot_dstc2.json` references to the *GoalOrientedBot* section of `server_config.json`. Therefore, `model_endpoint` parameter in `common_defaults` will be will be overridden with the same parameter from `model_defaults/GoalOrientedBot`.
Model argument names are provided as list in `model_args_names` parameter, where arguments order corresponds to component API.
When inferencing model via REST api, JSON payload keys should match component arguments names from `model_args_names`.
Default argument name for one argument components is *"context"*.
Here are POST requests examples for some of the library components:
| Component | POST request JSON payload example |
| --------- | -------------------- |
| **One argument components** |
| NER component | {"context":"Elon Musk launched his cherry Tesla roadster to the Mars orbit"} |
| Intent classification component | {"context":"I would like to go to a restaurant with Asian cuisine this evening"} |
| Automatic spelling correction component | {"context":"errror"} |
| Ranking component | {"context":"What is the average cost of life insurance services?"} |
| (Seq2seq) Goal-oriented bot | {"context":"Hello, can you help me to find and book a restaurant this evening?"} |
| **Two arguments components** |
| Question Answering component | {"context":"After 1765, growing philosophical and political differences strained the relationship between Great Britain and its colonies.", "question":"What strained the relationship between Great Britain and its colonies?"} |
Flasgger UI for API testing is provided on `<host>:<port>/apidocs` when running a component in `riseapi` mode.
# License
DeepPavlov is Apache 2.0 - licensed.
# Support and collaboration
If you have any questions, bug reports or feature requests, please feel free to post on our [Github Issues](https://github.com/deepmipt/DeepPavlov/issues) page. Please tag your issue with `bug`, `feature request`, or `question`. Also we’ll be glad to see your pull requests to add new datasets, models, embeddings, etc.
# The Team
DeepPavlov is built and maintained by [Neural Networks and Deep Learning Lab](https://mipt.ru/english/research/labs/neural-networks-and-deep-learning-lab) at [MIPT](https://mipt.ru/english/) within [iPavlov](http://ipavlov.ai/) project (part of [National Technology Initiative](https://asi.ru/eng/nti/)) and in partnership with [Sberbank](http://www.sberbank.com/).
<p align="center">
<img src="https://ipavlov.ai/img/ipavlov_footer.png" width="50%" height="50%"/>
</p>
Project details
Release history Release notifications | RSS feed
Download files
Download the file for your platform. If you're not sure which to choose, learn more about installing packages.
Source Distribution
deeppavlov-0.0.5.tar.gz
(208.9 kB
view hashes)